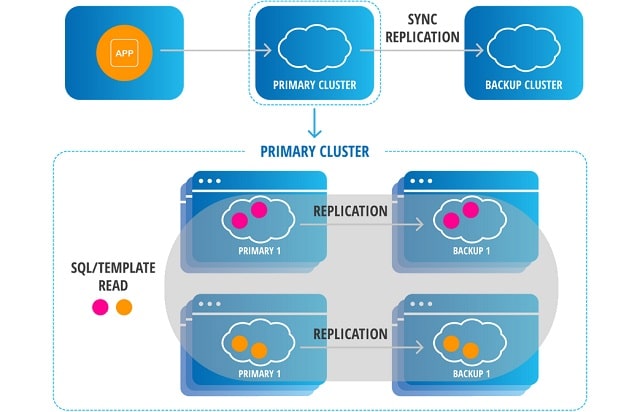
As businesses rely more and more on big data, accelerating applications becomes a critical issue so that companies can save time and money. It’s no surprise, then, that companies have been adopting in-memory computing through the years. In-memory computing platforms like data grids provide data processing speed and scalability required for business expansion. The future is digital—and organizations who delay a digital transformation or don’t consider an omnichannel approach are doomed to fail.
In-memory data grids are a cost-effective solution that does away with the complexity of disk- based databases. It’s a low-latency data fabric with high throughput that minimizes the need to access hard-disk-drive-based or solid-state-drive-based data storage. It reduces data movement over the network by collocating both the application and its data in the same memory space. In-memory data grids allow for easy scalability and acceleration of services.
Challenges Of Big Data
The huge amounts of data companies have to sift through can be a challenge, and organizations must choose alternative processing methods to get value out of them. Companies can either leverage big data for analytics or use them to enable new applications or products. Big data analytics helps in providing actionable insights previously impossible with traditional data processing methods. These insights are provided in real-time, allowing businesses to make sound decisions and react accordingly to crucial issues. This eliminates the need for sampling and moves to a more investigative approach to data analysis, which is a more effective method than simply running predetermined reports.
Another problem is that big data moves too fast and sometimes doesn’t fit specific database architectures. These fast-moving, huge amounts of data can’t be handled efficiently by traditional database management systems. Transitioning into an in-memory data grid will help in this regard, but this means that an ample amount of memory must be acquired, and RAM is still more expensive than disk memory so cost becomes a major part of the equation. This is the reason many companies prefer the best of both worlds: they keep frequently accessed data on disk and keep the rest in memory.
In-Memory Data Grids To The Rescue
Size and speed are the two main considerations in choosing a data storage and management infrastructure. Between these, the latter is the one that will tip the scales when it comes to making a decision between data infrastructure options.
One great argument for the in-memory data grid is that it can solve the problems mentioned earlier. In fact, an in-memory data grid is a common implementation for big data management because it uses a distributed cluster, which ensures availability, speed, and capacity. To be able to manage the massive amounts of data, in-memory data grids do their processing against the full dataset, more commonly referred to as “persistent store.” This capability allows the amount of data to exceed the amount of memory. Data can then be optimized to reside on both disk and memory. Systems with a persistent store capability also have the advantage of immediate performance even after a reboot. There’s no need to wait for the dataset to load into memory.
In-memory data grids distribute data so they can be stored on multiple servers, with each server operating in active mode. This allows organizations to add or reduce servers as dictated by the business. It also does away with tables and other traditional database features and uses an object-oriented, non-relational data model.
Networking and clustering capabilities, however, are the main attractions when it comes to in- memory data grids. These capabilities allow the infrastructure to provide features like failover, high availability, data replication, and data synchronization between clients. The cluster of servers acts as a hub of the infrastructure where applications connected to the cluster can share, replicate, and back up data using the cluster or another application.
Although big data has brought some challenges due to its potential complexity, it has also brought about some innovations in data processing—specifically, in-memory data grids. The challenge of big data is to rethink the ways we handle data and their practical applications. In- memory data grids address the challenges posed by big data: their processing power is 800 times faster than hard-disk drives, horizontal scalability through a distributed architecture addresses capacity limits, and reliability is ensured by a replication system that’s part of the data grid. As companies gather more data through the years, databases will reach a limit and no longer be able to handle the demand. The best—and in the future maybe the only—alternative is the in-memory data grid.